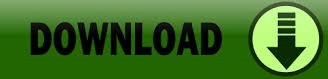

There are already some research results, such as the clustering of moving objects, the anomaly detection, the location and the prediction of movement trend, where the driving vehicle position prediction technology is continuously improved, but because of the theory and technology of the immature, most models can't be well adapted to the needs of moving vehicle position prediction.
HIDDEN MARKOV MODEL MATLAB CODE FORECASTING HOW TO
How to accurately predict the location information of the driving vehicle is a difficult problem needed to be solved collectively. In addition, the diversification of the moving vehicle environment also makes the problem more complex. However, during the time of two positioning information transmitted, the specific position information and the moving trajectory of the moving vehicle are not known. In general, the mobile vehicle periodically sends its position information to the central server. At present, the digital cities’ public transport vehicles, taxis and other vehicles are equipped with GPS and vehicle navigation equipment, which can be used to collect vehicle location information during different time periods, and connect, constitute a complete trajectory in a timed sequence, and then mine its dynamic trajectory. Meanwhile, vehicle trajectory prediction has gradually become one of the hot issues in the research. Therefore, Location Based Service (LBS) has been a hot issue of research that has been studied by domestic and foreign scholars. In many important application fields, such as intelligent transportation system (ITS), intelligent navigation, logistics distribution and mobile e-commerce, users all need to query and analyze the track position information of vehicles. Moreover, the time performance of DHMTP algorithm is also clearly improved compared with TPMO algorithm.Īs the rapid development of global location technology and mobile communication technology, mobile handheld devices such as mobile phones, PDA and mobile navigation systems are becoming more and more popular.

The experimental results demonstrate that the prediction accuracy of the proposed algorithm is increased by 18.3% compared with TPMO algorithm and increased by 23.1% compared with Naive algorithm in aspect of predicting the next k phases' trajectories, especially when traffic flow is greater, such as this time from weekday morning to evening. Finally, we propose a new algorithm (DHMTP) for vehicle trajectory prediction based on the hidden Markov model of double layers hidden states, and predict the nearest neighbor unit of location information of the next k stages. Secondly, we adopt Viterbi algorithm to seek the double layers hidden states sequences corresponding to the just driven trajectory.
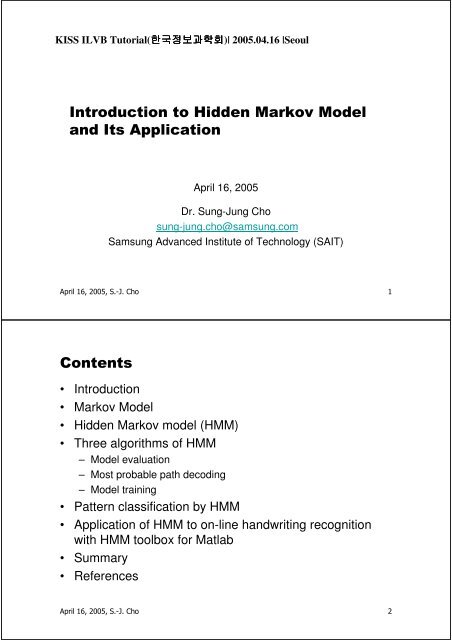
In this paper, firstly, we mine the double layers of hidden states of vehicle historical trajectories, and then determine the parameters of HMM (hidden Markov model) by historical data. Vehicle trajectory prediction can not only provide accurate location-based services, but also can monitor and predict traffic situation in advance, and then further recommend the optimal route for users. In Intelligent Transportation Systems (ITS), logistics distribution and mobile e-commerce, the real-time, accurate and reliable vehicle trajectory prediction has significant application value.
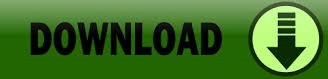